Dr. Muhammad Abid Dar
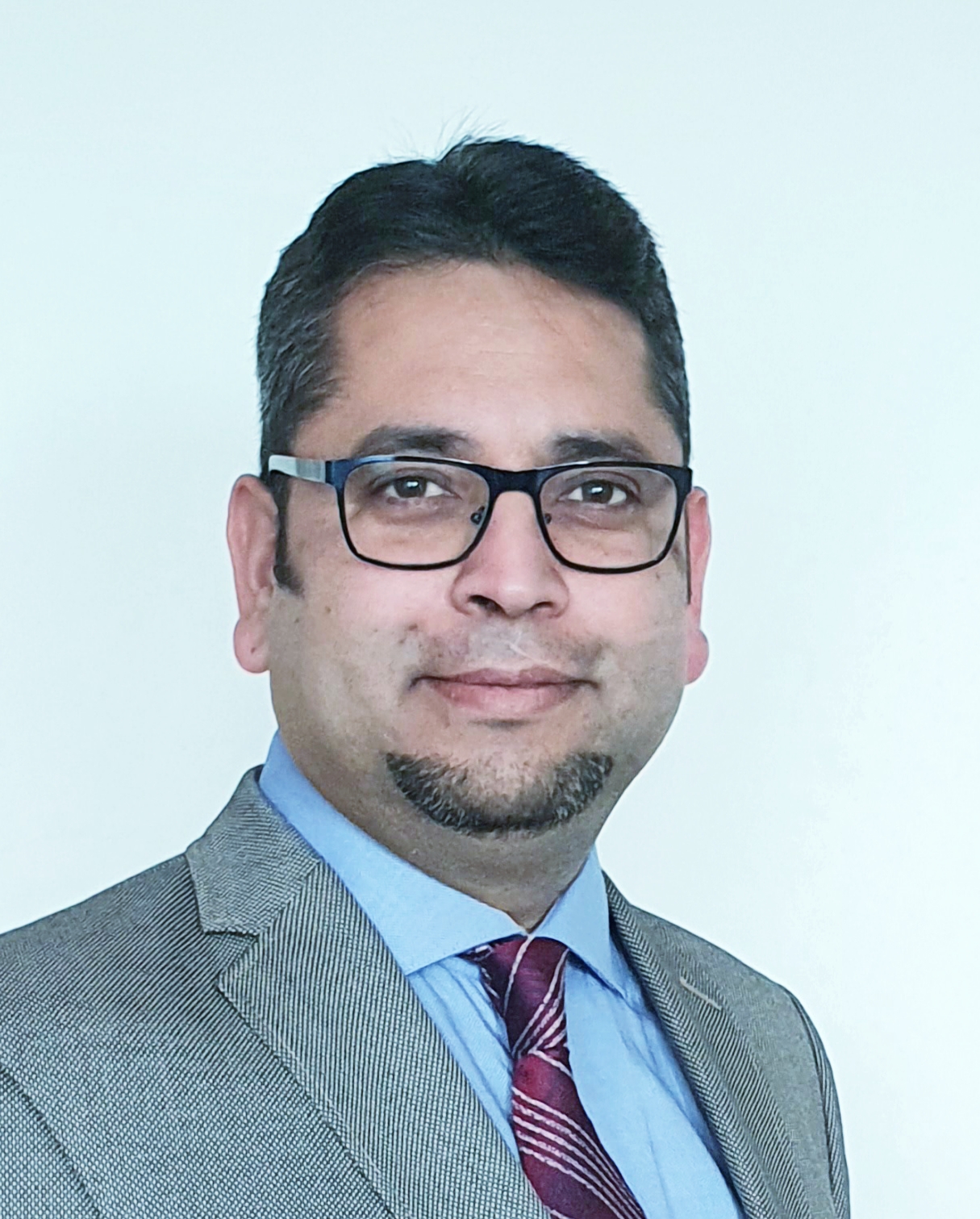
Dr. Muhammad Abid Dar is a Lecturer of Mathematics at the University of Gujrat, specializing in applied mathematics and discrete optimization. He earned his Ph.D. from University of Technology, Dresden (Germany) in 2020 and later did a one year post doc at the same university. Dr Dar has over 23 years of teaching experience in teaching mathematics courses in various institutes in Pakistan and abroad. His research focuses on discrete optimization, with publications in reputed peer-reviewed journals. His other areas of interest in research are mathematical didactic and STEM education. He is actively involved in curriculum development and STEM outreach. Outside of academia, he mentors young students in mathematics and enjoys engaging in science communication.
- Post Doc, Other
- Ph.D., Other
- M.Phil.,University of Gujrat
- M.Sc.,University of Punjab
- B.Sc.,University of Punjab
- F.Sc.,Gujranwala Board of Intermediate & Secondary Education
- Matric,Rawalpindi Board of Intermedia
- OVERSEAS SCHOLARSHIP FOR MS/MPhil LEADING TO PHD IN SELECTED FIELDS PHASE-II A merit based scholarship for a PhD from Germany
Student Name | Degree | Title | Status / Completed Year |
---|---|---|---|
Madiha Zakria | MS | REDUCTION ALGORITHMS TO TACKLE KIND-2 INSTANCE OF THE MINIMUM CONNECTIVITY INFERENCE PROBLEM At the nexus of several fields, discrete optimization is a significant area of applied mathematics that includes both theoretical and practical components. It deals with the issue of increasing or decreasing a function with respect to a set of requirements, often illuminating the spectrum of choices available to an individual in a particular situation. The Minimum Connectivity Inference problem (MCI) is also a discrete optimization problem, which is NP-hard. A simple, undirected, complete graph that includes a vertex set and an edge set serves as the foundation for this description. The MCI is a generalization of the Minimum Spanning Tree (MST). In the literature, there are two distinct kinds of MCI instances. The major objective of our research is to create alternative reduction strategies for kind-2 in order to find the faster solutions of the MCI problem. In this context, we generated kind-2 instances and then developed a reduction rule to simplify the instances while preserving their connectivity and optimality. | 2024 |
Maryam Saleem | MS | TO IMPROVE THE PERFORMANCE OF CASE BASE USING THE FREQUENT PATTERN-BASED FRAMEWORK This research investigates the impact of pre-processing on the transition of raw instances while ensuring that the functionality of the imitation agent remains unchanged. This study aims to conduct a comparative analysis of various algorithms and pre-processing approaches. Hence, it becomes possible to ascertain the most suitable algorithm for the case-based imitation system while employing a case-based approach to reasoning. Identifying the most analogous scenarios from a pool of previously documented examples is utilized to determine the optimal resolution for a given input issue. Reducing characteristics in a given scenario by eliminating non-useful ones can decrease the computational time required. This work utilizes Frequent pattern based algorithms as the chosen method for case selection. The current study proposes a novel approach utilizing an ordered case base incorporating L(1) frequent alphabetic preferences. This discussion will compare and contrast three key concepts: L(N ) item sets, common patterns, and ordered case bases. The case base was modified by replacing the matched cases with a singular case. The proposed algorithm aims to reduce the workload by incorporating past data. Pac-Man game has been taken as a data set for applying frequent pattern-based algorithms. Our proposed FP-based algorithm has reduced the number of features while enhancing efficiency and accuracy. | 2024 |
Ayesha Firdous | MS | THE MINIMUM CONNECTIVITY INFERENCE PROBLEM: PRE-PROCESSING OF KIND-2 INSTANCES In mathematics, discrete optimization plays a crucial role in finding the optimal solution from a finite set of possibilities, ensuring efficiency and effectiveness in decision-making processes often involving integer or combinatorial variables. It has plethora of applications in various fields such as network design, routing problems, transportation scheduling, biology, finance, portfolio optimization, and machine learning. The Minimum Connectivity Inference problem is also a discrete optimization problem, which is N P-hard and is a generalization of the well known Minimum Spanning Tree problem. It involves examining a simple, complete, and undirected graph with a set of vertices and predefined subsets of these vertices. Its purpose is to identify a minimum set of edges that ensures the presence of a spanning tree for every individual cluster. In literature, there are two kinds of problem instances related to the MCI problem. The classical MCI issue, also known as the kind-1 instance, has at least the same number of clusters as vertices. The other category is the kind-2 instances, which has more vertices than clusters. While, literature mostly consists of preprocessing of instances of kind-1, we developed an exact reduction rule to simplify the kind-2 instances of the MCI problem. Using this rule we can downsize an MCI-instance while preserving their connectedness and optimality, with the ultimategoal of finding the most efficient and optimal solution in polynomial time. Importantly, this reduction rule is also suitable for instances of kind-1. Our research achieves better reductions of kind-2 instances of the MCI problem, leading to a significant reductions. | 2024 |
Um-e-Aiman | MS | NEW INSTANCE REDUCTION RULES FOR THE MINIMUM CONNECTIVITY INFERENCE INSTANCES OF KIND-1 The Minimum Connectivity Inference (MCI) is an N P-hard problem of discrete optimization. Given an undirected, complete graph and countably many subsets (clusters) of its set of vertices, the MCI problem is to determine an optimal edge subset such that each cluster is connected with regard to this optimal subset. The MCI problem is an NP-hard problem due to its large size of instances, making it difficult to solve in polynomial time. The prime objective of our research is to find different reduction techniques for the MCI problem to reduce its complexity and minimize its solution time. For this, we introduce five new instance reduction rules for the MCI problem instances of kind-1. In these rules, we remove both the vertices and the clusters from the original instances and get the reduced instances of the MCI problem, which are easy to solve for further algorithms. To check the effectiveness of the new rules, we use the numerical experiments. | 2023 |
Attiq-ur-Rehman | MS | A ROUGH SET-BASED FRAMEWORK FOR CASE-BASED DATA REDUCTION IN VIRTUAL WORLD This research investigates the influence of pre-processing on the case-based imitationperformance of an imitation agent while ensuring that the imitation performance is not compromised. To achieve this, a rough set-based case selection approach is utilized to minimize the time required to search the case base with a minimal number of cases. Subsequently, a pre-processing technique is proposed to decrease the time required to search the case base while simultaneously maintaining diversity with minimal cases. The impact of this proposed technique on the case-based imitation performance of an imitation agent in a simulation game environment is then evaluated. Furthermore, the usual seven measures of similarity are compared to identify the optimal measures of similarity for the selected video game. Insights regarding the impact of methods that involve pre-processing on the imitation performance of the agent are provided. In conclusion, the proposed pre-processing techniques have the potential to enhance the imitation performance of the case-based imitation agent. | 2023 |
Sara Liaqat | MS | THE MINIMUM CONNECTIVITY INFERENCE INSTANCES OF KIND-1: NEW DATA REDUCTION RULES TO REMOVE VERTICES The field of discrete optimization encompasses a tremendous amount of research. Inaddition to that, it discusses the development of the contemporary information system. The majority of discrete optimization problems are notoriously challenging to resolve, sometimes known as being NP -hard. The phrase Non-Deterministic Polynomial hard is what is meant to be understood by the term NP -hard. The Minimum Connectivity Inference (MCI) problem is an example of a discrete optimization topic that has NP-hard complexity. It has been investigated in a wide variety of fields under a variety of categories. The primary objective of our research is to come up with the most effective response to the MCI. In order to accomplish this, we discussed fresh data reduction ideas and test algorithms through the use of computational simulations and numerical experiments. | 2023 |
Usman Asghar | MS | USE OF MULTI-CUT APPROACH IN CONSTRAINT GENERATION ALGORITHM FOR THE MINIMUM CONNECTIVITY INFERENCE PROBLEM The Minimum Connectivity Inference (MCI) problem is studied in this researchwork. The MCI problem is one of the computationally challenging problems, which iscategorized as N P-hard problem. The class of N P-hard problems poses a great chal-lenge to the scientific community. Finding the exact solution of the MCI problem issometimes impossible for the instances of moderate to large size. In this regard, an exact solution setup known as Constraint Generation Algorithm (CGA) has beenrecently introduced in the literature. The scheme CGA, which works iteratively, usescut-set formulation of the MCI problem to pick useful constraints of its ILP. However,in this study we focus the more powerful modeling framework known as multi-cut formulation. We prove the strength of multi-cut formulation over the cut-set one. Moreover, the detailed algorithmic implementation of the proposed and existing CGA is discussed as well. | 2023 |
- Selected member of Board of Studies for Mathematics department in University of Gujrat (2007-10)
- Elected member of Academic Council for University of Gujrat
- Selected member of Board of Studies for Mathematics department in 2021
-
1. Muhammad Abid Dar, Andreas Fischer, John Martinovic, Guntram Scheithauer “Integer linear programming formulations for the minimum connectivity inference problem and model reduction principles” Discrete Optimization, February 2021 DOI: doi.org/10.1007/978-3-030-02487-1_7
-
2. Muhammad Abid Dar, Andreas Fischer, John Martinovic, Guntram Scheithauer “An improved flow-based formulation and reduction principles for the minimum connectivity inference problem” Optimization, April 2018 DOI:
-
1. Muhammad Abid DarAndreas Fischer, John Martinovic, Guntram Scheithauer “A Computational Study of Reduction Techniques for the Minimum Connectivity Inference Problem” Advances in Mathematical Methods and High Performance Computing , February 2019 _DOI: doi.org/10.1007/978-3-030-02487-1_7