Naveed Anwer Butt
Specialization : Artificial Intelligence
Email : [email protected]
Office Number : +(92) 533643375Office Exten : 176
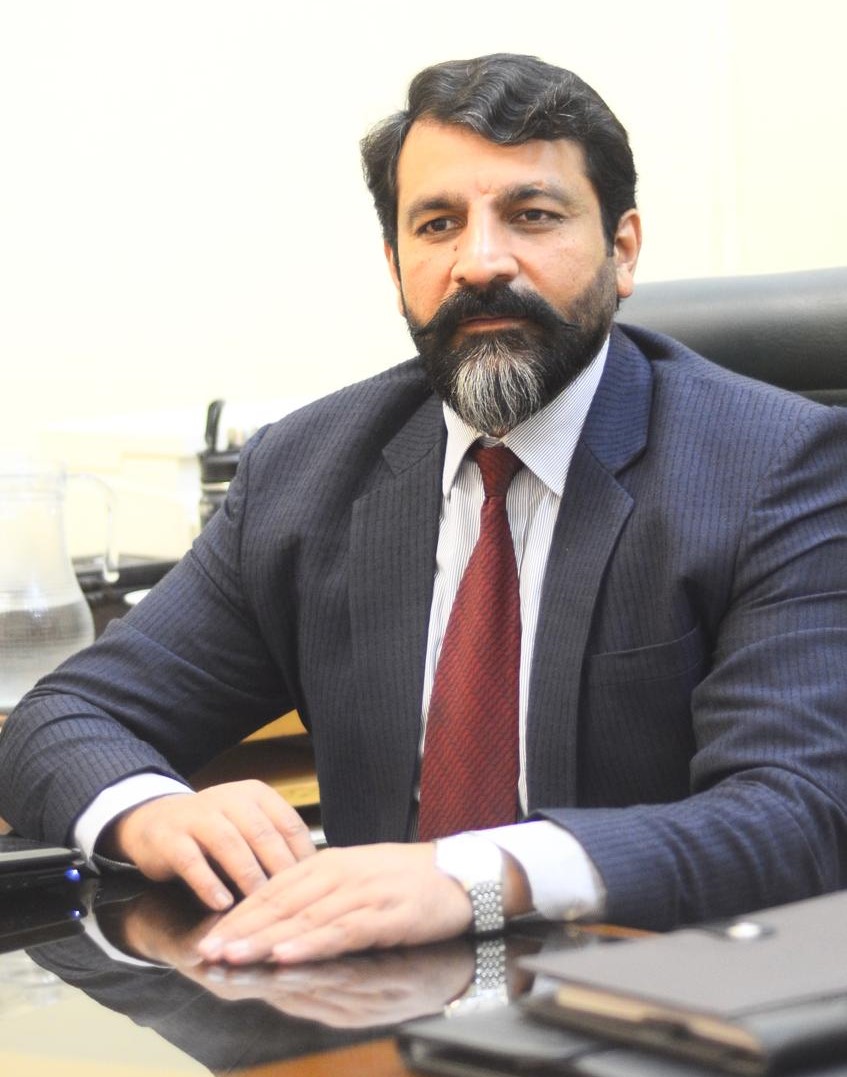
I am Assistant Professor in the Department of Computer Science, Faculty of Computing & Information Technology, University of Gujrat since 2007. My research work and teaching career spans over 25 years in various Organization and HEC Recognized Universities and Institutes. My research interests include Artificial Intelligence, ML/DL, Neuroscience, Behavioral Data Modeling & Prescriptive Analytics, Explority Data Analytics, Data Mining, Opinion Mining and Process Mining. With extensive experience across diverse environments and disciplines, I have developed a strong ability to collaborate effectively with individuals from varied backgrounds. My career has honed my skills in being self-reliant, analytical, adaptable, and resilient. I possess the ability to work independently with a task focused approach, while maintaining high levels of organization and professionalism. Whether working as part of a team or individually, I strive to deliver innovative and impactful solutions to complex problems.
- Ph.D. Computer Science,International Islamic University
- MS Computer Science,International Islamic University
- M.Sc. Computer Science,University of Agricultural Faisalabad
- B.Sc.,University of Punjab
- Training of Trainers Programme on Academia-Industry Linkage conducted by University of Gujrat & UNIDO in partnership with Entrepreneurship and small & Medium Enterprise Centre, LUMS- United Nations Industrial Development Organization under (UNIDO) CLUSTER DEVELOPMENT PROGRAMME PAKISTAN
- Certification of Programming with Java 2 Enterprise Edition (J2EE) APTECH Computer Education World Wide
- One day Training Workshop on ADDS Data Mining Tool conducted by Center for Agro-Informatics Research & FAST & National ICT R&D Fund.
- Certification of Programming with MS.NET APTECH Computer Education World Wide
- Evaluation and Assessment in Semester Systems conducted by HEC
- Member of Association for Computing Machinery (ACM) , 2018.
- Convener of Departmental Review and Research Committee (DRRC) for MPhil and Ph.D. Rules of Department of Computer Science, University of Gujrat, Pakistan. , University of Gujrat, Pakistan.
- Member of Board of Faculty (BOF) of Department of Computer Science , University of Gujrat, Pakistan.
- Convener of Board of Studies (BOS) of Department of Computer Science , University of Gujrat, Pakistan.
- Member of Academic Council, University of Gujrat, Pakistan.
- Convener and Member of Departmental Accreditation Council of Computer Science for National Computing Education Accreditation Council (NCEAC).
- Member of IEEE, Institute of Electrical and Electronics Engineers , 2018
- Member, American Society of Mechanical , 2008
Student Name | Degree | Title | Status / Completed Year |
---|---|---|---|
Atifa Arooj | MS | Classification of Schizophrenia Disease Using Machine and Deep Learning Identification of mental illnesses, such as schizophrenia is very significant but still a challenging problem. Schizophrenia is one of the most concerned severe and chronic disorders of the brain that affects the ability of the brain to distinguish between what is real and unreal. In the past decade, different artificial intelligence techniques have been introduced to assist mental health providers, but it is still a time taking, challenging, and critical task to identify schizophrenia. Moreover, it is very hard to validate the signal generated from the areas of the brain, and the big challenge is to find the location of brain diseases. Due to slow BOLD response, fMRI is unable to perform adequate temporal sampling. On the other side, EEG offers high temporal resolution but with poor localization of signal sources. Integration of these technologies allows to overcome the limitation of both and gives a new research direction. Our objective is to identify the schizophrenia disease through different machine learning algorithms such as K-Nearest Neighbor, Neural Network, Support Vector Machine, Gaussian Processes, Random Forest, Ada Boost, Quadratic Discriminant Analysis, Decision Tree, Naive Bayes Classifier, Radial basis function Support Vector Machine and deep learning algorithm such as Convolutional Neural Network to classify the schizophrenia disease by applying appropriate filters of deep convolutional neural networks and to identify the location of schizophrenia disease using fMRI and EEG simultaneously. Our system takes advantage of existing previous work and identifies schizophrenia with higher accuracy which is a great improvement in this field. | 2021 |
Amna Akhter | MS | Lungs Cancer Detection Using Bronchoscopy images with image Segmentation and Hybrid Feature Approach Lung cancer is a disease which leads a patient to death if not diagnosed accurately on time. CT lung image segmentation is a vital introductory advance for lung image investigation. Region extraction is important for localization of region of interest for several applications. For region of interest, Gross Tumour Volume (GTV) contouring is important method. SOM Pixel Labelling with Reduce Cluster membership and Deterministic Feature Clustering (SPLRCM&DFC) needed improvement in FCM. We have performed segmentation through FCM. Secondly, the images have been skull stripped along FCM, so region of interest become more prominent. At third, we have matched both FCM and Skull Removal of FCM. To identify the significance and variation of intensities, automatic segmentation of low intensities, features must be well selected. In our research work, Connected Component for Tumour Volume Extraction CCTVE is run for calculating area of connected components. Segmentation Method with Improved Fuzzy Clustering Mean (FCM) is used for classification. For significant region extraction, a new method Improved Volume is achieved which is identifying lungs tumour significantly and constantly. For better feature selection Combination of Features for Classification of Tumour CFCT is applied. More than 80% accuracy is achieved and thirteen features are combined to classify tumorous and non-tumorous images. For checking of result when we have compared both, therefore Dice overlapped index is 98 % and JI is 96 %. | 2021 |
Kiran Shahzadi | MS | A Deep Learning based framework for Imitating Human-like Play in Virtual World In recent years’ Artificial intelligence has focused on imitation of human behaviour. People were also involved in learning actions and behavior in the future. In addition, a major rise in demand for smart agents who can mimic characteristics of video games and human players has increased the qualities of amusement and contents. The agents that are produced using most of the current methods are however, viewed as mechanical, contributing to dissatisfaction and furthermore, lack of dedication. In order to achieve more credible and accurate reproduction, the studies indicate an imitation learning framework. Two learning paradigms, namely deep learning and machine learning were explored in this proposed context to construct a computational model. We used two case studies Pacman and PUBG in this research. The first one is used to predict human behaviour and second is used to validate the imitation power of our model. This study used various deep learning variants in order to mimic the actions of human players. In addition, the Recurrent Neural Network(RNN), Gated Recurrent Unit(GRU) and LSTM were used to find the optimal deep learning algorithm. The result demonstrated that recurrent neural network is overall best with an average accuracy 99.96% along with machine learning Xgboost algorithm with average accuracy 96.31%. To compare the performance of the models we used mean square error, root mean square error, and accuracy. Two statistical methods, Mann-Whitney U Test and Cosine Similarity Test were also used to measure the believability. With high accuracy both techniques validate the reproduced observed behaviour. In comparison to the other methods, a deep learning method preferably tackles the issue in the form of prediction and efficient imitation of human actions. | 2021 |
Huda Gull | MS | A Multi-Prefecture Study Applying Multivariate Approaches for Predicting and Demystifying Weather Data Variations Affect Covid-19 Spread At the end of December 2019, a lot of cases of pneumonia, later named covid-19 spread across the different countries by the means of people traveling from China. Now the whole world is suffering from this alarming Pandemic including Pakistan. As it provides the rich source of exploring the variants that affect the covid-19 contagion. The covid-19 spread in Pakistan so, the association between covid-19 cases and weather parameters be assessed. The dataset includes the weather parameters (Temperature, Humidity, Windspeed, and dewpoints) and covid-19 cases form March 2020 to February 2021 for Pakistan provinces and Islamabad. The proposed study implemented multivariate techniques to demystifying the weather parameters impact on the covid-19 contagion. The following multivariate approaches were used: Linear Multiple Regression, Linear Stepwise Regression, Multivariate Adaptive Regression Splines, and Loess Regression. For the detailed analysis, implement the Spline Curve regression model. To validate the splines curve regression model results, the machine learning regressors are implemented. The performance metrics RMSE, MSE, MAE, and R2 used to measure the performance of the machine learning models and spline curve. The proposed experiments showed that the weather parameters are salient feature to predict the covid-19 spread. The temperature and humidity do affect the spread of covid-19. The spline curve results showed during the 1-wave for all the provinces temperature has positive relationship with daily confirmed cases and for 2-wave the temperature has negative correlation with daily confirmed cases rather Baluchistan. Another conclusion from the experiment is that humidity has a negative association with mortality and confirmed cases in all provinces when applied to the datasets from March 2020 to February 2021. As a result, an increase in humidity reduces covid-19 spread and lowers the death rate. The Loess Regression and Multivariate Adaptive Regression splines are performed well, the performance of multivariate approaches measured with RMSE performance metric. | 2021 |
Ayesha Javaid | MS | Human Behavior Modeling Through AI Based Approaches Human behavior prediction has been the subject of interest since the start of mankind. Human being were always interested in finding out the future actions and behavior. They are different from each other; they behave differently in different situations yet the studies show that human behavior is not random so there is still hope. Human behavior is normally dependent on their previous experiences so we can expect that they might behave the same as they did in similar conditions previously. Neural networks are based on human cognition process, and serve as a useful tool to predict future actions. We used multiple neural network functions to predict future actions of human beings. The Generalized regression based model was the best found model, it was later used to build a controller agent that could act in place of a human. The results depicted that controller agents are capable enough to replace human beings only if we can overcome the problems of premature convergence and local minima. | 2014 |
Khawer Shakeel | MS | Educational Data Mining to Analyze Student’s Learning and Evaluation Behaviors Using Ensemble Classification Educational Data Mining (EDM) is a topical region these days with many areas to be researched. It supports in decision making by applying Data Mining (DM) techniques on education related data to deal with matters that would be inflexible without them. These techniques try to extract valuable patterns that may lead to strategic policy making and to determine the behaviors of both, the teacher and student from educational point of view. Therefore, this evidence will lead us on the way to the finding of which approaches must be avoided, which and how teaching tactics can be improved to each group of students or to expect which students will perform good or poor so that specific group of students can be facilitated timely on initial stage. Administration might rapidly be capable to practice this innovative information as directions for course redesign and as indication for executing new assessments criteria. The main purpose of my study was to find out the some useful arrays of information from university environment answering some question about identification of weak students needing more help, categorizing the poor performance areas of students where some necessary actions need to be taken, finding the difference in evaluation in changed surroundings and to evaluate the way in which students are evaluated by teachers , also to detect that the how the job status of teacher correlate with students performance. Technically, the hot topic for the researchers today is “Whether a combined classifier model provides improved performance than the best between the base level classifiers”. This main question is tried to be discuss in this thesis. It also describes some classification techniques of different style of working like probability, decision tree and artificial neural network based as base classifiers and some ensemble classification methods based on integration of algorithms to generate more certain and accurate results. The advantages of one classification method over other also debated, and finally some academic suggestions, useful models for calculated policy making to improve the efficiency of studying, are also proposed. | 2014 |
- Founding Head of Project management Office (PMO) Faculty of Computing &Information Technology. (2008 - 2011)
- Founding Head of CS&IT Research Cell , Faculty of Computing &Information Technology (2008 - 2011).
- 1. Naveed Anwer Butt, Zafar Mahmood “The Development of Intelligent Agents: A case-based reasoning approach to achieve human-like peculiarities via Playback of Human Traces” Ieee Access, May 2023 DOI:
- 2. Naveed Anwer Butt, Huda Gull “A multi-prefecture study applying multivariate approaches for predicting and demystifying weather data variations affect COVID-19 spread” Information Systems And E-Business Management, May 2023 DOI:
- 3. Naveed Anwer, Zaffar Mehmood “Behavioral and Performance Analysis of a Real-Time Case Study Event Log: A Process Mining Approach” Applied Sciences-Basel, March 2023 DOI:
- 4. Ghani Ur Rehman, Naveed Anwer Butt “Performance Evaluation and Comparison of Cooperative Frameworks for IoT-Based VDTN” Sustainability, March 2023 DOI:
- 5. Zafar Mahmood, Naveed Anwer Butt “Efficient Scheduling of Home Energy Management Controller (HEMC) Using Heuristic Optimization Techniques” Sustainability, January 2023 DOI:
- 6. Zafar Mahmood , Naveed Anwer Butt “Generation of Controlled Synthetic Samples and Impact of Hyper-Tuning Parameters to Effectively Classify the Complex Structure of Overlapping Region” Applied Sciences-Basel, August 2022 DOI:
- 7. Naveed Anwer Butt, Mian Muhammad Awais, and Qamar Abbas “Improved Diagnostic Accuracy in Dependent Personality Disorders A Comparative Study of Neural Architectures and Hybrid Approaches on Functional Magnetic Resonance Imaging Data” Journal Of Medical Imaging And Health Informatics, August 2019 DOI:
- 8. Muhammad Touseef IKRAM, , Naveed Anwer BUTT , Muhammad Tanvir AFZAL “Open source software adoption evaluation through feature level sentiment analysis using Twitter dat” Turkish Journal Of Electrical Engineering And Computer Sciences, June 2016 DOI:
- 9. Muhammad Touseef IKRAM, Muhammad Tanvir AFZAL , Naveed Anwer BUTT “Open source software adoption evaluation through feature level sentiment analysis using Twitter data” Turkish Journal Of Electrical Engineering And Computer Sciences, June 2016 DOI:
- 10. Syed Hassan , Naveed Anwer, Jungwon Yoon “Open architecture dynamic manipulator design philosophy (DMD)” Robotics And Computer-Integrated Manufacturing, September 2008 DOI:
-
1. Aneela Mazhar, Naveed Anwer Butt, Ayesha Javed Butt “Do We Really Have to Consider Data Mining Techniques for Meteorological Data-2015” 2015 Fourth International Conference on Aerospace Science and Engineering (ICASE), September 2015 _DOI: 10.1109/ICASE.2015.7489525
-
2. Khawar Shakeel, Naveed Anwer Butt “Educational Data Mining to Reduce Student Dropout Rate by Using Classification” International Conference on Big Data Analysis and Data Mining, May 2015 _DOI: 20.31199/JCOMPUT.2015.876576
-
3. Ayesha Javed Butt , Naveed Anwer Butt “Predicting Mobile Subscriber’s Behavior from Contextual Information Extraction: SMS Data” 21st International Conference on Neural Information Processing (ICONIP 2014) , Part III, Springer International Publishing Switzerland, LNCS 8836, pp. 661–672, 3 - 6 November, 2014, November 2014 _DOI: 10.1007/978-3-319-12637-1
-
4. Ayesha Javed Butt , NaveedAnwer Butt “The Soar of Cognitive Architecture” CTITDubai 2013) 3rd International Conference on Current Trends in Information Technology, Dubai Volume 3 Pages: 135- 142, December 2013, December 2013 _DOI: 10.1109/CTIT.2013.6749492
-
5. Naveed Anwer , Aneela Mazhar “Measuring Weather Prediction Accuracy Using Sugeno Based Adaptive Neuro Fuzzy Inference System” NCM2012: 2012 8th International Conference on Networked Computing and Advanced Information Management, Souel, Korea, Volume 1 Pages:214-219, ISBN: 978-1-4673-0893-9, 24 – 26 April 2012, April 2012 _DOI: 12945103
-
6. Naveed Anwer, Ayesha Rashid “Feature based Opinion Mining of Online Customer Reviews Using Frequency Distribution and Bayesian Statistics” NCM2010:2010 6th international Conference on Networked Computing and Advanced Information Management. , Souel, Korea Volume 1 Pages:57-62, 2010, August 2010 _DOI: 11530876